Big Data Analytics In Banking Market Size, Share, Industry Trends and Forecast to 2033
This report provides an in-depth analysis of the Big Data Analytics in Banking market, covering trends, forecasts, and market sizing from 2023 to 2033. Insights include industry analysis, segmentation, regional performance, and notable technology advancements impacting the sector.
Metric | Value |
---|---|
Study Period | 2023 - 2033 |
2023 Market Size | $7.50 Billion |
CAGR (2023-2033) | 12.4% |
2033 Market Size | $25.35 Billion |
Top Companies | IBM, SAS Institute, SAP, Microsoft, Oracle |
Last Modified Date | 15 Nov 2024 |
Big Data Analytics In Banking Market Report (2023 - 2033)
Big Data Analytics In Banking Market Overview
What is the Market Size & CAGR of Big Data Analytics In Banking market in 2023?
Big Data Analytics In Banking Industry Analysis
Big Data Analytics In Banking Market Segmentation and Scope
Request a custom research report for industry.
Big Data Analytics In Banking Market Analysis Report by Region
Europe Big Data Analytics In Banking Market Report:
Europe is expected to experience considerable growth from $2.59 billion in 2023 to $8.77 billion by 2033. The rising need to comply with GDPR and other regulatory frameworks is driving demand for big data analytics in banking across the region.Asia Pacific Big Data Analytics In Banking Market Report:
The Asia Pacific region is witnessing significant growth, with the market size in 2023 projected at $1.24 billion, expanding to $4.19 billion by 2033. Rapid digitalization and the adoption of innovative banking solutions are key drivers, along with the increasing number of tech-savvy consumers.North America Big Data Analytics In Banking Market Report:
North America holds a substantial share of the market, with a projected size of $2.55 billion in 2023, growing to $8.61 billion by 2033. The region benefits from advanced technological adoption and stringent regulations necessitating robust data analytics solutions for compliance and risk management.South America Big Data Analytics In Banking Market Report:
The South American market is relatively nascent, valued at $0.29 billion in 2023, with expectations to reach $0.98 billion by 2033. The region's growth is bolstered by improving infrastructure and the increasing focus on enhancing financial services through data analytics.Middle East & Africa Big Data Analytics In Banking Market Report:
The Middle East and Africa's market is estimated at $0.83 billion for 2023 and is projected to grow to $2.79 billion by 2033. Increasing investments in IT infrastructure and the proliferation of fintech companies in the region are significant growth drivers.Request a custom research report for industry.
Big Data Analytics In Banking Market Analysis By Technology
Global Big Data Analytics in Banking Market, By Technology Market Analysis (2023 - 2033)
In the banking sector, various technologies drive data analytics, leading to improved decision-making. Data warehousing accounts for a lion's share of the market, standing at $5.15 billion in 2023 with a notable growth projection to $17.42 billion by 2033. Analytics platforms and data integration tools are also essential, ensuring effective data processing and usage.
Big Data Analytics In Banking Market Analysis By Application
Global Big Data Analytics in Banking Market, By Application Market Analysis (2023 - 2033)
Applications of big data analytics in banking include risk management ($4.12 billion in 2023, projected to reach $13.93 billion by 2033), fraud detection ($1.65 billion in 2023, anticipated growth to $5.59 billion), and customer analytics ($0.81 billion in 2023, aiming for $2.74 billion). Each application significantly enhances operational efficiency and customer satisfaction.
Big Data Analytics In Banking Market Analysis By Deployment Mode
Global Big Data Analytics in Banking Market, By Deployment Mode Market Analysis (2023 - 2033)
The deployment of big data analytics technologies can be categorized into on-premises and cloud-based solutions. Currently, on-premises solutions dominate the market, generating $6.42 billion in 2023 and rising to $21.70 billion by 2033, while cloud-based solutions are expected to grow from $1.08 billion to $3.65 billion.
Big Data Analytics In Banking Market Analysis By End User
Global Big Data Analytics in Banking Market, By End-User Industry Market Analysis (2023 - 2033)
Commercial banks represent the largest segment in the market, valued at $5.15 billion in 2023 and expecting to expand to $17.42 billion. Other significant contributors include investment banks and credit unions, with sizes of $2.02 billion and $0.32 billion respectively for 2023, anticipated to grow further by 2033.
Big Data Analytics In Banking Market Analysis By Data Source
Global Big Data Analytics in Banking Market, By Data Source Market Analysis (2023 - 2033)
Key data sources utilized in big data analytics in banking encompass transaction data, which commands a sizable market share of $5.15 billion in 2023, social media data valued at $2.02 billion, and customer data expected to see growth from $0.32 billion. These data sources greatly influence analytics outcomes and business strategies.
Big Data Analytics In Banking Market Trends and Future Forecast
Request a custom research report for industry.
Global Market Leaders and Top Companies in Big Data Analytics In Banking Industry
IBM:
IBM is a pioneering technology company leading in AI and big data analytics, providing robust solutions tailored for the banking sector to enhance operational efficiency and compliance.SAS Institute:
SAS offers advanced analytics software and solutions that empower banks to leverage data for smarter decisions, especially in risk management and fraud detection.SAP:
SAP integrates analytics into banking products, helping institutions streamline operations and improve customer service through data insights.Microsoft:
Microsoft provides comprehensive cloud-based solutions with strong analytics capabilities, enabling financial institutions to harness big data for enhanced decision-making.Oracle:
Oracle specializes in database software and cloud solutions, facilitating effective data management and analytics in the banking sector.We're grateful to work with incredible clients.
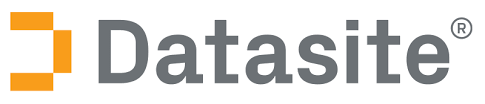
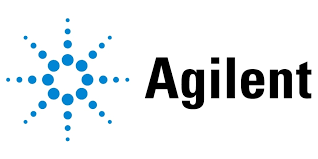

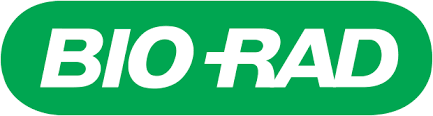
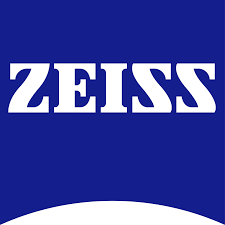
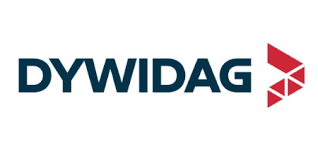
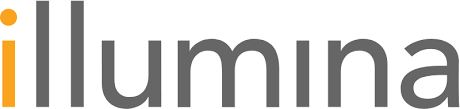
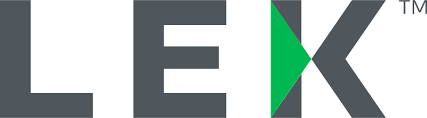
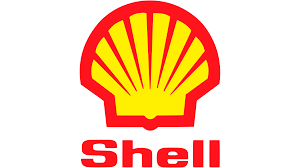