Big Data Analytics In Retail Market Size, Share, Industry Trends and Forecast to 2033
This report provides a comprehensive analysis of the Big Data Analytics in Retail market from 2023 to 2033, offering insights into market trends, regional dynamics, technological advancements, and forecasts to understand future opportunities and challenges.
Metric | Value |
---|---|
Study Period | 2023 - 2033 |
2023 Market Size | $10.50 Billion |
CAGR (2023-2033) | 12% |
2033 Market Size | $34.16 Billion |
Top Companies | IBM, Oracle, SAS Institute, SAP |
Last Modified Date | 15 Nov 2024 |
Big Data Analytics In Retail Market Report (2023 - 2033)
Big Data Analytics In Retail Market Overview
What is the Market Size & CAGR of Big Data Analytics In Retail market in 2023?
Big Data Analytics In Retail Industry Analysis
Big Data Analytics In Retail Market Segmentation and Scope
Request a custom research report for industry.
Big Data Analytics In Retail Market Analysis Report by Region
Europe Big Data Analytics In Retail Market Report:
The European market is projected to rise from $2.84 billion in 2023 to $9.23 billion by 2033. This growth is spurred by heightened competition and regulatory compliance requirements, compelling retailers to adopt data analytics solutions.Asia Pacific Big Data Analytics In Retail Market Report:
In Asia Pacific, the market is anticipated to grow from $2.28 billion in 2023 to $7.43 billion by 2033, driven by rapid digitalization and a growing middle class. Retailers are increasingly adopting big data analytics to cater to diverse consumer preferences and improve operational efficiencies.North America Big Data Analytics In Retail Market Report:
North America remains a dominant player, with the market growing from $3.57 billion in 2023 to approximately $11.62 billion by 2033. The region is characterized by significant investments in advanced analytics technologies by both large and medium-sized retailers.South America Big Data Analytics In Retail Market Report:
The South American market is smaller but expected to expand from $0.37 billion in 2023 to $1.20 billion in 2033. This growth is fueled by increasing internet penetration and the rise of e-commerce platforms seeking insights to better serve their consumers.Middle East & Africa Big Data Analytics In Retail Market Report:
The market in the Middle East and Africa is set to increase from $1.44 billion in 2023 to $4.69 billion by 2033. Factors like urbanization and growing smartphone adoption are facilitating the adoption of big data analytics in retail.Request a custom research report for industry.
Big Data Analytics In Retail Market Analysis By Analytics Type
Global Big Data Analytics in Retail Market, By Analytics Type Market Analysis (2023 - 2033)
Descriptive analytics dominates the market with a size of $6.68 billion in 2023 and is expected to reach $21.74 billion by 2033, holding a steady share of 63.65%. Predictive analytics follows closely, with growth from $2.72 billion to $8.83 billion, making up 25.86% of the market. Prescriptive analytics is also gaining traction, with a projected market size of $1.10 billion in 2023 to $3.58 billion by 2033, showing its rising importance in decision-making processes.
Big Data Analytics In Retail Market Analysis By Application
Global Big Data Analytics in Retail Market, By Application Market Analysis (2023 - 2033)
The customer analytics segment holds a significant portion of the market, valued at $6.68 billion in 2023. This segment is expected to experience growth up to $21.74 billion by 2033, reflecting the critical need for retailers to understand customer behavior and preferences. Supply chain analytics follows, projected to increase from $2.72 billion to $8.83 billion during the same period, emphasizing inventory management and logistical efficiency.
Big Data Analytics In Retail Market Analysis By Deployment Model
Global Big Data Analytics in Retail Market, By Deployment Model Market Analysis (2023 - 2033)
Cloud-based solutions are the cornerstone of the market, expected to grow from $8.58 billion in 2023 to $27.93 billion by 2033. On-premises solutions account for a smaller segment, projected to expand from $1.92 billion to $6.23 billion, as businesses balance between cloud advantages and local control.
Big Data Analytics In Retail Market Analysis By End User
Global Big Data Analytics in Retail Market, By End-User Market Analysis (2023 - 2033)
Large retailers command the market with a size of $8.58 billion in 2023 and anticipated growth to $27.93 billion by 2033, capturing 81.76% market share. Small and medium retailers, while accounting for a smaller segment of $1.92 billion, are projected to grow to $6.23 billion, representing a significant opportunity for analytics providers.
Big Data Analytics In Retail Market Analysis By Technology
Global Big Data Analytics in Retail Market, By Technology Market Analysis (2023 - 2033)
Technologically, market leaders are focusing on integrating AI and machine learning within analytics frameworks. The use of big data tools is expected to rise from $6.68 billion in 2023 to $21.74 billion by 2033, displaying a 63.65% share. Data visualization tools, while currently smaller, are also expected to show strong growth as businesses seek to present analytics findings in actionable formats.
Big Data Analytics In Retail Market Trends and Future Forecast
Request a custom research report for industry.
Global Market Leaders and Top Companies in Big Data Analytics In Retail Industry
IBM:
IBM offers a comprehensive suite of analytics solutions tailored for retail, enabling companies to harness big data for operational efficiency and enhanced customer service.Oracle:
Oracle provides a robust set of cloud-based analytics tools designed to help retailers gain deep insights into consumer behaviors and market trends.SAS Institute:
SAS specializes in advanced analytics and business intelligence solutions that empower retailers to analyze large datasets effectively.SAP:
SAP delivers analytics solutions that integrate seamlessly with retail operations, offering real-time insights for competitive edge.We're grateful to work with incredible clients.
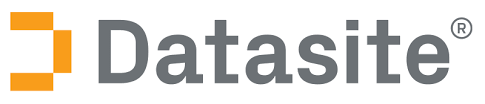
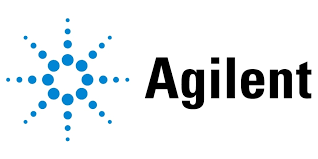

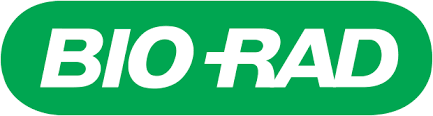
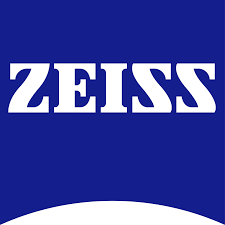
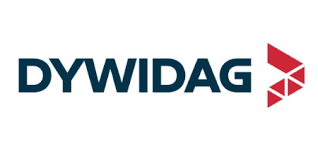
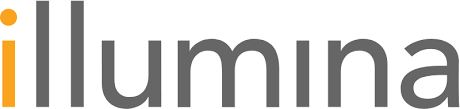
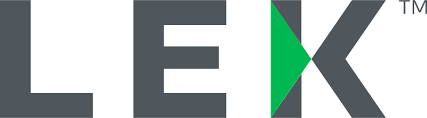
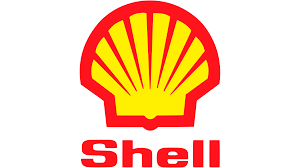