Content Recommendation Engine Market Size, Share, Industry Trends and Forecast to 2033
This report provides an in-depth analysis of the Content Recommendation Engine market, covering market size, trends, segmentation, and forecasts from 2023 to 2033.
Metric | Value |
---|---|
Study Period | 2023 - 2033 |
2023 Market Size | $5.60 Billion |
CAGR (2023-2033) | 12.5% |
2033 Market Size | $19.11 Billion |
Top Companies | Amazon Web Services, Google Cloud, IBM Watson, Adobe, Microsoft Azure |
Last Modified Date | Invalid Date |
Content Recommendation Engine Market Report (2023 - 2033)
Content Recommendation Engine Market Overview
What is the Market Size & CAGR of Content Recommendation Engine market in 2023?
Content Recommendation Engine Industry Analysis
Content Recommendation Engine Market Segmentation and Scope
Request a custom research report for industry.
Content Recommendation Engine Market Analysis Report by Region
Europe Content Recommendation Engine Market Report:
In Europe, the market size was estimated at $2.06 billion in 2023, which is expected to reach $7.04 billion by 2033, driven by increasing demand for personalized marketing solutions and stringent GDPR regulations enhancing data protection.Asia Pacific Content Recommendation Engine Market Report:
In 2023, the Asia Pacific region holds a market value of $0.91 billion, projected to grow to $3.12 billion by 2033. The growth is driven by the rapid adoption of digital technologies and increasing internet penetration, particularly in countries like China and India.North America Content Recommendation Engine Market Report:
North America, the leading region in this market, has a market size of $1.84 billion in 2023, projected to grow to $6.29 billion by 2033. The strong presence of major tech companies and continuous investment in AI technologies are key growth drivers.South America Content Recommendation Engine Market Report:
The South American market is valued at $0.19 billion in 2023, with expectations to reach $0.64 billion by 2033. The growth is fueled by rising mobile usage and a burgeoning digital economy.Middle East & Africa Content Recommendation Engine Market Report:
The Middle East and Africa market was valued at $0.59 billion in 2023 and is forecasted to grow to $2.02 billion by 2033. An increase in e-commerce and digital transformation initiatives are propelling this growth.Request a custom research report for industry.
Content Recommendation Engine Market Analysis By Type
Global Content Recommendation Engine Market, By Type Market Analysis (2024 - 2033)
The market analysis by type highlights three main categories: Collaborative Filtering, Content-Based Filtering, and Hybrid Systems. Collaborative Filtering dominated the market with a size of $3.69 billion in 2023, projected to rise to $12.58 billion by 2033, holding 65.84% market share. Content-Based Filtering, valued at $1.14 billion in 2023, is anticipated to reach $3.87 billion by 2033, capturing 20.28% share. Hybrid Systems, which combine both approaches, had a size of $0.78 billion in 2023, expected to reach $2.65 billion by 2033, holding 13.88% market share.
Content Recommendation Engine Market Analysis By Industry
Global Content Recommendation Engine Market, By Industry Market Analysis (2024 - 2033)
In the industry segmentation, the major sectors utilizing recommendation engines include E-commerce, Media and Entertainment, Education, News and Publication, and Social Media. E-commerce leads with a market size of $2.40 billion in 2023, expected to grow to $8.19 billion by 2033, representing a share of 42.84%. Media and Entertainment segments are valued at $1.26 billion, forecasted to reach $4.30 billion by 2033, with a share of 22.52%. Education, News and Publications, and Social Media have notable growth contributions, showcasing the versatile application of content recommendation engines across different industries.
Content Recommendation Engine Market Analysis By Technology
Global Content Recommendation Engine Market, By Technology Market Analysis (2024 - 2033)
Technological advancements significantly impact the Content Recommendation Engine market, with key segments including Machine Learning, Natural Language Processing, and Big Data. Machine Learning currently leads with a market size of $3.69 billion in 2023, projected to grow to $12.58 billion by 2033, capturing 65.84% of the market share, demonstrating its critical role in developing intelligent recommendation systems. Natural Language Processing and Big Data also show remarkable growth, indicating a strong trend towards systems that better understand contextual user needs.
Content Recommendation Engine Market Analysis By Deployment
Global Content Recommendation Engine Market, By Deployment Market Analysis (2024 - 2033)
Deployment models in the Content Recommendation Engine market include Cloud-Based, On-Premises, and Hybrid Deployment. The Cloud-Based segment leads with a market size of $3.69 billion in 2023, set to rise to $12.58 billion by 2033, maintaining a significant 65.84% share. The On-Premises segment has a market size of $1.14 billion, projected to grow to $3.87 billion, with a share of 20.28%. Hybrid Deployment also shows potential growth, reflecting the flexibility required by businesses in terms of infrastructure and data security.
Content Recommendation Engine Market Analysis By User
Global Content Recommendation Engine Market, By User Type Market Analysis (2024 - 2033)
User type segmentation focuses on Businesses, End-Users, and Developers. Businesses account for the largest market share with a size of $3.69 billion in 2023, expected to reach $12.58 billion by 2033, holding 65.84% share. End-Users are valued at $1.14 billion in 2023, forecasted to expand to $3.87 billion, representing 20.28% of the market share. Developers also play a key role in shaping recommendation systems, projected to grow from $0.78 billion to $2.65 billion by 2033, making up 13.88% market share.
Content Recommendation Engine Market Trends and Future Forecast
Request a custom research report for industry.
Global Market Leaders and Top Companies in Content Recommendation Engine Industry
Amazon Web Services:
AWS offers a suite of machine learning services, including personalized recommendation systems that help businesses enhance user engagement and drive sales.Google Cloud:
Google’s recommendation engine utilizes user data and AI models to deliver personalized content across various platforms, from e-commerce to social media.IBM Watson:
IBM's Watson provides advanced analytics and machine learning capabilities for businesses seeking to enable smarter content recommendations.Adobe:
Adobe offers AI-driven marketing solutions that include content recommendation engines, allowing brands to optimize user experience.Microsoft Azure:
Azure provides powerful tools for developing AI-based recommendation systems that help businesses increase their operational efficiency.We're grateful to work with incredible clients.
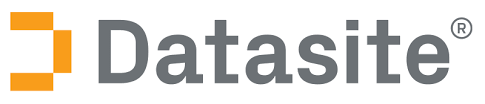
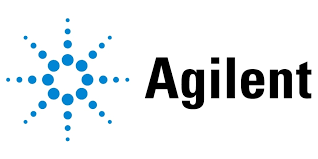

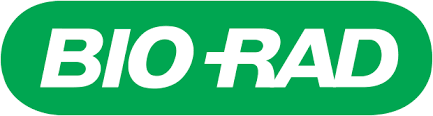
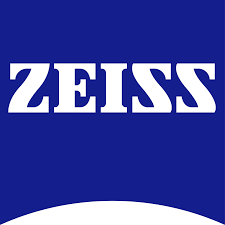
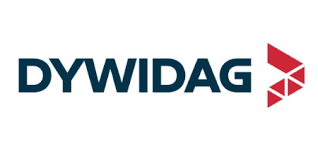
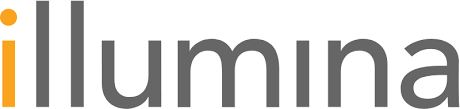
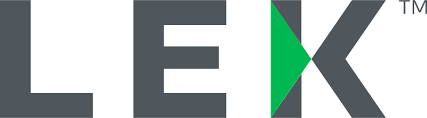
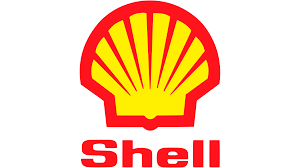
Related Industries
FAQs
What is the market size of content Recommendation Engine?
The global market size for the Content Recommendation Engine is projected to reach approximately $5.6 billion by 2033, growing at a compound annual growth rate (CAGR) of 12.5% from its 2023 value.
What are the key market players or companies in this content Recommendation Engine industry?
Key players in the Content Recommendation Engine industry include leading technology firms that specialize in machine learning algorithms, data analytics, and AI-driven solutions, actively shaping the competitive landscape.
What are the primary factors driving the growth in the content Recommendation Engine industry?
The growth of the Content Recommendation Engine industry is driven by the increasing demand for personalized content delivery, advancements in artificial intelligence, and the rising importance of user engagement across digital platforms.
Which region is the fastest Growing in the content Recommendation Engine?
The Asia Pacific region is currently the fastest-growing market for Content Recommendation Engines, expected to increase from $0.91 billion in 2023 to $3.12 billion by 2033.
Does ConsaInsights provide customized market report data for the content Recommendation Engine industry?
Yes, ConsaInsights offers customized market report data tailored to the specific needs of clients in the Content Recommendation Engine industry, ensuring comprehensive insights.
What deliverables can I expect from this content Recommendation Engine market research project?
Deliverables from the Content Recommendation Engine market research project include detailed market analysis reports, segmentation insights, key trends, forecasts, and competitive landscape assessments.
What are the market trends of content Recommendation Engine?
Current market trends in the Content Recommendation Engine field include increased utilization of machine learning, emphasis on personalized user experiences, and a shift towards hybrid deployment models.