Deep Learning Market Size, Share, Industry Trends and Forecast to 2033
This report provides a comprehensive analysis of the Deep Learning market from 2023 to 2033, highlighting key trends, market size, growth forecasts, and major players in the industry. It offers insights into various segments, regional performance, and future prospects.
Metric | Value |
---|---|
Study Period | 2023 - 2033 |
2023 Market Size | $43.30 Billion |
CAGR (2023-2033) | 39.7% |
2033 Market Size | $1560.82 Billion |
Top Companies | NVIDIA Corporation, Google LLC, IBM Corporation, Microsoft Corporation, Amazon Web Services (AWS) |
Last Modified Date | 15 Nov 2024 |
Deep Learning Market Report (2023 - 2033)
Deep Learning Market Overview
What is the Market Size & CAGR of Deep Learning market in 2023?
Deep Learning Industry Analysis
Deep Learning Market Segmentation and Scope
Request a custom research report for industry.
Deep Learning Market Analysis Report by Region
Europe Deep Learning Market Report:
The European market is projected to rise from $12.70 billion in 2023 to $457.79 billion by 2033. Regulatory frameworks promoting technological advancement and strong industrial capabilities in countries like Germany and France foster a conducive environment for growth.Asia Pacific Deep Learning Market Report:
The Asia Pacific region, projected to grow from $8.61 billion in 2023 to $310.45 billion by 2033, is characterized by significant investment in AI research and infrastructure improvements, particularly in countries like China and India. The proliferation of digital technologies and an increasing number of tech startups contribute to this growth.North America Deep Learning Market Report:
North America remains a leader in the Deep Learning market, with values jumping from $15.41 billion in 2023 to $555.50 billion by 2033. The region's dominance stems from a concentration of tech companies and significant investment in research and development.South America Deep Learning Market Report:
In South America, the Deep Learning market is expected to grow from $4.28 billion in 2023 to $154.36 billion by 2033. Adoption of digital transformation is gaining traction, with sectors engaging in advanced data analytics and AI-driven insights.Middle East & Africa Deep Learning Market Report:
The Middle East and Africa are emerging markets with expectations to grow from $2.29 billion in 2023 to $82.72 billion by 2033, driven by increasing investment in smart city initiatives and digital transformation across various sectors.Request a custom research report for industry.
Deep Learning Market Analysis By Architecture
Global Deep Learning Market, By Architecture Market Analysis (2023 - 2033)
The architecture segment reflects a growing investment in advanced models. Feedforward Neural Networks and Convolutional Neural Networks (CNN) are projected to see substantial growth due to their applications in data classification and image processing, respectively. Transformer models, known for their efficiency in natural language tasks, are also contributing significantly to market dynamics.
Deep Learning Market Analysis By Industry
Global Deep Learning Market, By Industry Market Analysis (2023 - 2033)
In healthcare, the market is anticipated to grow from $23.11 billion to $833.16 billion between 2023 and 2033, indicating strong demand for AI in diagnostics and personalized medicine. The automotive industry's investment in autonomous systems positions it for growth from $10.79 billion to $388.80 billion in the same period, while finance and retail are equally focusing on leveraging these technologies for enhanced customer experiences and risk management.
Deep Learning Market Analysis By Deployment Type
Global Deep Learning Market, By Deployment Type Market Analysis (2023 - 2033)
Cloud-Based solutions dominate the deployment type segment, expected to grow from $26.19 billion in 2023 to $943.98 billion by 2033, driven by the increasing shift towards cloud computing. On-Premises deployments, while still significant, will grow at a more moderate pace due to concerns over data privacy and security.
Deep Learning Market Analysis By Application
Global Deep Learning Market, By Application Market Analysis (2023 - 2033)
The application segment indicates strong growth potential in image recognition and natural language processing, which are projected to witness significant increases in demand due to their integration into consumer products and business solutions. Speech recognition technology is also poised for growth as businesses integrate voice interfaces into services.
Deep Learning Market Analysis By Technology
Global Deep Learning Market, By Technology Market Analysis (2023 - 2033)
The market displays a variety of technologies with significant advancements in frameworks and hardware acceleration, projected to drive innovation. The continuous evolution of AI frameworks is enabling developers to create more sophisticated applications, while hardware acceleration solutions are enhancing processing efficiency.
Deep Learning Market Trends and Future Forecast
Request a custom research report for industry.
Global Market Leaders and Top Companies in Deep Learning Industry
NVIDIA Corporation:
A leader in GPU technologies, NVIDIA plays a crucial role in deep learning by providing the high-performance computing power required for training complex models.Google LLC:
Google's TensorFlow framework has become synonymous with deep learning development, showcasing the company's commitment to AI advancements.IBM Corporation:
IBM leverages its extensive experience in AI and blockchain technology, driving innovations through Watson AI solutions.Microsoft Corporation:
With Azure AI, Microsoft is actively providing tools and services for businesses to implement deep learning solutions efficiently.Amazon Web Services (AWS):
AWS offers a plethora of AI services that facilitate the deployment of deep learning models, making them a significant player in this market.We're grateful to work with incredible clients.
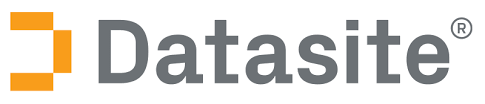
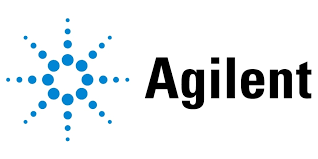

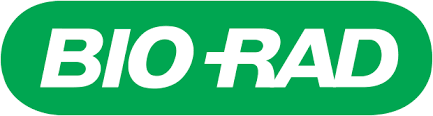
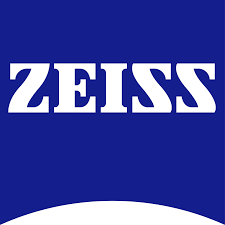
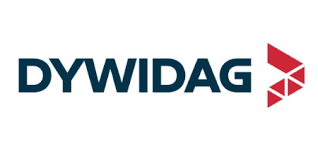
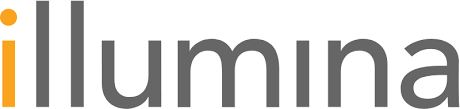
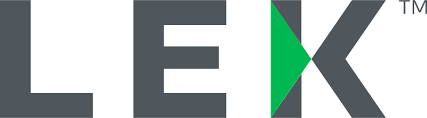
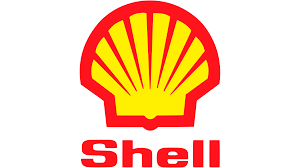