Federated Learning Solutions Market Size, Share, Industry Trends and Forecast to 2033
This report offers a comprehensive analysis of the Federated Learning Solutions market, including trends, market size, regional insights, and forecasts from 2023 to 2033, aiming to guide stakeholders in decision-making processes.
Metric | Value |
---|---|
Study Period | 2023 - 2033 |
2023 Market Size | $5.20 Billion |
CAGR (2023-2033) | 12% |
2033 Market Size | $16.92 Billion |
Top Companies | Google AI, IBM, NVIDIA, Microsoft |
Last Modified Date | 15 Nov 2024 |
Federated Learning Solutions Market Report (2023 - 2033)
Federated Learning Solutions Market Overview
What is the Market Size & CAGR of Federated Learning Solutions market in 2023?
Federated Learning Solutions Industry Analysis
Federated Learning Solutions Market Segmentation and Scope
Request a custom research report for industry.
Federated Learning Solutions Market Analysis Report by Region
Europe Federated Learning Solutions Market Report:
Europe's Federated Learning Solutions market was valued at $1.74 billion in 2023, projected to grow to $5.65 billion by 2033. The region's stringent data protection laws, such as GDPR, are central to the increasing demand for federated learning technologies. Moreover, European firms are actively seeking innovative solutions for collaborative machine learning without compromising user privacy.Asia Pacific Federated Learning Solutions Market Report:
The Asia Pacific region is witnessing rapid growth in the Federated Learning Solutions market, valued at $0.92 billion in 2023 and projected to reach $3.00 billion by 2033. The adoption of AI and machine learning technologies, particularly in countries like China and India, is driving this growth. Moreover, governmental initiatives promoting data privacy and the utilization of local data for technological advancements further contribute to the regional market's expansion.North America Federated Learning Solutions Market Report:
North America leads the global market for Federated Learning Solutions, with a current market size of $1.77 billion projected to increase to $5.76 billion by 2033. The region's emphasis on technological advancements, coupled with considerable investment from both public and private sectors, acts as a catalyst for market growth. Additionally, the increasing concerns over data security and privacy regulations are pushing enterprises to adopt federated learning.South America Federated Learning Solutions Market Report:
The South American market, though smaller, is gaining traction, with a size of $0.50 billion in 2023 and expected to grow to $1.62 billion by 2033. Growth is propelled by increasing digitization efforts and expanding interest in innovative data management solutions across various industries, including agriculture and finance.Middle East & Africa Federated Learning Solutions Market Report:
The Middle East and Africa region is anticipated to see steady growth in the Federated Learning Solutions market, from $0.27 billion in 2023 to $0.88 billion by 2033. Growing awareness of the importance of data privacy, coupled with technological advancements in AI, is expected to enhance federated learning adoption in various industries across this region.Request a custom research report for industry.
Federated Learning Solutions Market Analysis By Use Case
Global Federated Learning Solutions Market, By Use Case Market Analysis (2023 - 2033)
The federated learning solutions market is highly influenced by various use cases, reflecting significant investments in machine learning across health, finance, and automotive sectors. For instance, in healthcare, federated learning facilitates safer data sharing for patient records while complying with regulations. In finance, it aids in fraud detection without exposing sensitive customer information.
Federated Learning Solutions Market Analysis By Architecture
Global Federated Learning Solutions Market, By Architecture Market Analysis (2023 - 2033)
In terms of architecture, federated learning can be categorized into centralized and decentralized models. The centralized approach is more prevalent, accounting for substantial market share due to its efficiency in managing large-scale data analysis. However, decentralized models are gaining attention for their advantages in scalability and data sovereignty.
Federated Learning Solutions Market Analysis By Technology
Global Federated Learning Solutions Market, By Technology Market Analysis (2023 - 2033)
Technological advancements such as secure multi-party computation and differential privacy are instrumental in enhancing federated learning solutions. Companies are focusing on integrating these technologies to ensure security, aiding in building trust amongst users while utilizing federated learning.
Federated Learning Solutions Market Analysis By Industry
Global Federated Learning Solutions Market, By Industry Market Analysis (2023 - 2033)
Industries like healthcare, automotive, and finance are the primary sectors driving the federated learning solutions market. Specifically, healthcare is leading with a market share of 66.8% in 2023 and anticipated to maintain dominance in the next decade, highlighting the critical role of data privacy and compliance in patient care.
Federated Learning Solutions Market Trends and Future Forecast
Request a custom research report for industry.
Global Market Leaders and Top Companies in Federated Learning Solutions Industry
Google AI:
Google AI has made significant contributions to the development of federated learning frameworks, enhancing collaborative machine learning while ensuring data privacy.IBM:
IBM invests heavily in federated learning to improve its Watson AI services, focusing on data privacy and interoperability within the healthcare sector.NVIDIA:
NVIDIA is advancing federated learning applications through its high-performance AI hardware, facilitating real-time data processing without compromising security.Microsoft:
Microsoft is leveraging federated learning in its Azure cloud services, allowing enterprises to build and deploy AI models efficiently while protecting sensitive data.We're grateful to work with incredible clients.
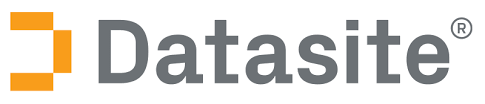
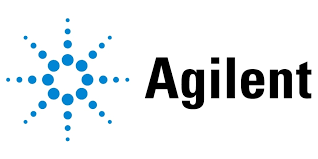

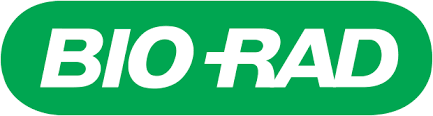
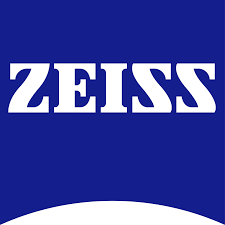
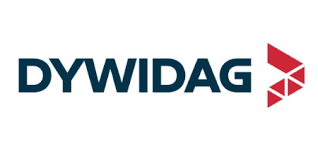
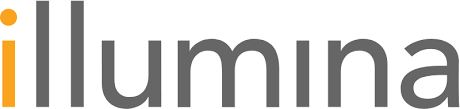
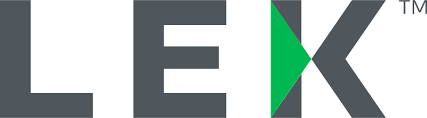
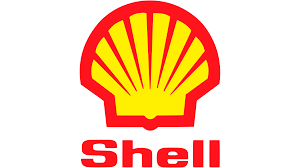