Machine Learning As A Service Market Size, Share, Industry Trends and Forecast to 2033
This report provides an in-depth analysis of the Machine Learning as a Service (MLaaS) market from 2023 to 2033, covering market dynamics, size, segmentation, regional insights, key players, and future trends.
Metric | Value |
---|---|
Study Period | 2023 - 2033 |
2023 Market Size | $5.60 Billion |
CAGR (2023-2033) | 12.3% |
2033 Market Size | $18.75 Billion |
Top Companies | IBM, Google, Microsoft, Amazon, Alibaba |
Last Modified Date | 15 Nov 2024 |
Machine Learning As A Service Market Report (2023 - 2033)
Machine Learning As A Service Market Overview
What is the Market Size & CAGR of Machine Learning As A Service market in 2023 and 2033?
Machine Learning As A Service Industry Analysis
Machine Learning As A Service Market Segmentation and Scope
Request a custom research report for industry.
Machine Learning As A Service Market Analysis Report by Region
Europe Machine Learning As A Service Market Report:
In Europe, the MLaaS market size is estimated at USD 1.64 billion in 2023, anticipated to grow to USD 5.48 billion by 2033. The region's emphasis on innovation and regulatory compliance is driving demand for customized ML services across various industries.Asia Pacific Machine Learning As A Service Market Report:
In 2023, the MLaaS market in the Asia Pacific region is valued at USD 1.10 billion and is expected to grow to USD 3.68 billion by 2033, indicating a substantial shift as businesses increasingly embrace digital transformation strategies. Countries like China and India are leading in market adoption, driven by rapid advancements in AI technologies and increasing investments from tech giants.North America Machine Learning As A Service Market Report:
North America leads the MLaaS market, with a valuation of USD 2.01 billion in 2023, expected to grow to USD 6.73 billion by 2033. The region boasts high adoption rates driven by the presence of major technology firms, innovative startups, and robust infrastructure enhancing machine learning capabilities.South America Machine Learning As A Service Market Report:
The South American market for MLaaS was valued at USD 0.51 billion in 2023, projected to reach USD 1.71 billion by 2033. The growth is fueled by the rising penetration of cloud computing across the region and the mounting need for data analytics solutions.Middle East & Africa Machine Learning As A Service Market Report:
The Middle East and Africa's MLaaS market is relatively nascent, valued at USD 0.34 billion in 2023 but expected to expand to USD 1.14 billion by 2033. Increased investments in the digital transformation of enterprises and heightened interest in AI applications are key growth drivers.Request a custom research report for industry.
Machine Learning As A Service Market Analysis By Service Type
Global Machine Learning as a Service Market, By Service Type Market Analysis (2023 - 2033)
The MLaaS market, segmented by service type, consists of model development, model deployment, and consulting services. Model development accounts for a substantial share of the market, projected to grow from USD 3.49 billion in 2023 to USD 11.67 billion by 2033, representing 62.25% of the market share. Model deployment and consulting services are significant as well, with their respective growth reflecting the increasing complexity of machine learning applications across various sectors.
Machine Learning As A Service Market Analysis By Application
Global Machine Learning as a Service Market, By Application Market Analysis (2023 - 2033)
Applications of MLaaS span several domains including customer service, predictive analytics, risk management, and marketing. Each application is critical in leveraging machine learning capabilities to optimize operational functionalities. Customer service, for instance, is poised for substantial growth, showing its importance in enhancing user experience, with an expected significant share in the future market dynamics.
Machine Learning As A Service Market Analysis By Industry
Global Machine Learning as a Service Market, By Industry Market Analysis (2023 - 2033)
The MLaaS market's industrial segmentation includes BFSI, retail, healthcare, manufacturing, and others. The BFSI sector is rapidly adopting ML solutions for risk assessment and fraud detection while healthcare is integrating machine learning for diagnostics and patient care improvement. The retail industry utilizes MLaaS to enhance customer experience and optimize inventory management.
Machine Learning As A Service Market Analysis By Deployment Model
Global Machine Learning as a Service Market, By Deployment Model Market Analysis (2023 - 2033)
Deployment models comprising public, private, and hybrid cloud environments direct the MLaaS buying behavior among organizations. Public cloud services dominate due to affordability and scalability, representing a major segment of market growth while hybrid models are gaining traction as organizations seek balanced control over their data.
Machine Learning As A Service Market Analysis By End User
Global Machine Learning as a Service Market, By End-User Market Analysis (2023 - 2033)
Segments of end-users include small and medium businesses and large enterprises, with SMEs showing a significant share due to the ability to leverage MLaaS for cost savings and operational efficiency.
Machine Learning As A Service Market Trends and Future Forecast
Request a custom research report for industry.
Global Market Leaders and Top Companies in Machine Learning As A Service Industry
IBM:
IBM offers a comprehensive MLaaS platform, leveraging its extensive cloud computing capabilities to bring advanced analytics and AI to enterprises.Google:
Google Cloud's MLaaS solutions provide users with robust tools that support model development and deployment tailored for various applications.Microsoft:
Microsoft's Azure MLaaS empowers businesses to develop machine learning models efficiently, offering tools that facilitate data access and collaboration.Amazon:
Amazon Web Services (AWS) provides a rich suite of MLaaS functionalities, focusing on scalability and integration with existing cloud architectures.Alibaba:
Alibaba Cloud delivers tailored machine learning services suited for businesses across e-commerce, finance, and other sectors, enhancing decision-making.We're grateful to work with incredible clients.
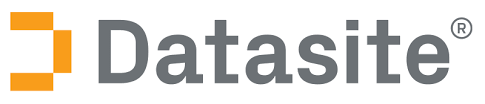
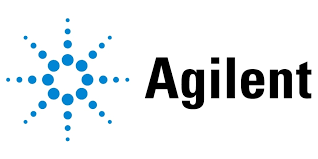

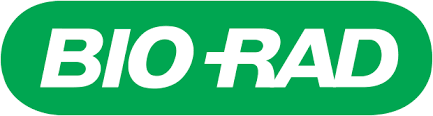
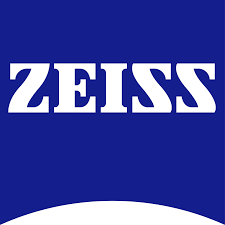
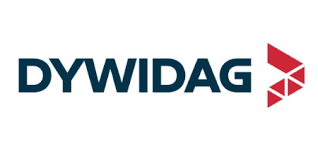
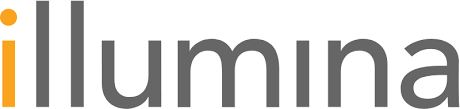
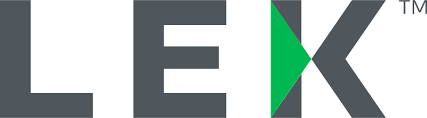
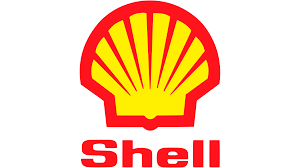