Machine Learning Market Size, Share, Industry Trends and Forecast to 2033
This report offers a comprehensive analysis of the Machine Learning market from 2023 to 2033, covering market size, growth projections, competitive landscape, and key trends driving the industry.
Metric | Value |
---|---|
Study Period | 2023 - 2033 |
2023 Market Size | $27.50 Billion |
CAGR (2023-2033) | 8.5% |
2033 Market Size | $63.83 Billion |
Top Companies | Google, IBM, Microsoft, Amazon, NVIDIA |
Last Modified Date | 15 November 2024 |
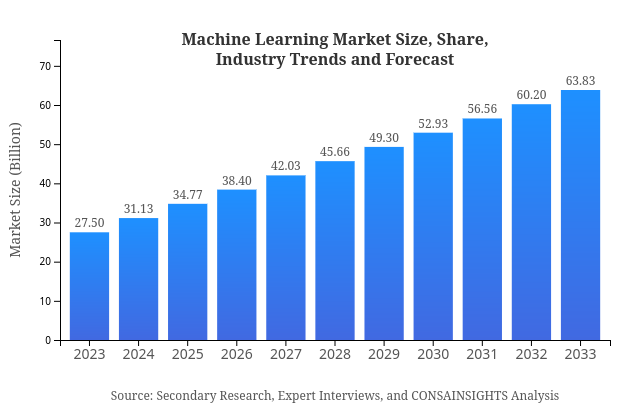
Machine Learning Market Overview
What is the Market Size & CAGR of Machine Learning market in 2023?
Machine Learning Industry Analysis
Machine Learning Market Segmentation and Scope
Request a custom research report for industry.
Machine Learning Market Analysis Report by Region
Europe Machine Learning Market Report:
In Europe, the Machine Learning market is expected to scale from $6.83 billion in 2023 to $15.86 billion by 2033. The region benefits from a well-established tech ecosystem and significant investments in AI research and development.Asia Pacific Machine Learning Market Report:
In the Asia Pacific region, the Machine Learning market was valued at $5.94 billion in 2023 and is expected to reach $13.78 billion by 2033, showcasing a robust growth trajectory driven by burgeoning technological adoption and government initiatives promoting AI technologies.North America Machine Learning Market Report:
North America leads the market with a valuation of $10.56 billion in 2023, expected to surge to $24.50 billion by 2033. The presence of key market players and high technological adoption rates underpin this region's dominance.South America Machine Learning Market Report:
The South American market has recorded a value of $2.22 billion in 2023, with projections indicating growth to $5.16 billion by 2033. This growth is fueled by increasing investments in technology and infrastructure.Middle East & Africa Machine Learning Market Report:
The market in the Middle East and Africa is valued at $1.95 billion in 2023, projected to grow to $4.53 billion by 2033. The emerging digital economy and interest in AI solutions are key drivers of this growth.Request a custom research report for industry.
Machine Learning Market Analysis By Use Case
Global Machine Learning Market, By Use Case Market Analysis (2024 - 2033)
The healthcare segment dominates the Machine Learning market, expected to grow from $18.37 billion in 2023 to $42.63 billion by 2033, accounting for approximately 67% market share. Financial services follow, projected to expand from $7.40 billion to $17.18 billion, capturing around 27% market share. Retail application remains significant but at a smaller market capacity, anticipated to grow from $1.73 billion to $4.01 billion.
Machine Learning Market Analysis By Technology
Global Machine Learning Market, By Technology Market Analysis (2024 - 2033)
Key technologies in this market include predictive analytics, image recognition, and natural language processing. Predictive analytics remains the leader at $18.37 billion in 2023 and growing to $42.63 billion by 2033. Image recognition and natural language processing are also gaining traction, with respective market sizes of $7.40 billion and $1.73 billion in 2023.
Machine Learning Market Analysis By Industry
Global Machine Learning Market, By Industry Market Analysis (2024 - 2033)
Industries have increasingly adopted Machine Learning for operational efficiency. Healthcare is the predominant sector, leveraging data for better patient care and operational performance. Financial services utilize Machine Learning for fraud detection, while the retail sector focuses on personalized customer experiences through data analysis.
Machine Learning Market Analysis By Deployment
Global Machine Learning Market, By Deployment Market Analysis (2024 - 2033)
In terms of deployment, cloud-based solutions lead the market due to their scalability and cost-effectiveness, expected to grow from $23.17 billion in 2023 to $53.78 billion by 2033. On-premises solutions also hold a share, but they are less favored among enterprises due to the higher costs and maintenance requirements.
Machine Learning Market Analysis By Organization Size
Global Machine Learning Market, By Organization Size Market Analysis (2024 - 2033)
Small and Medium-sized Enterprises (SMEs) dominate the Machine Learning market with a size of $23.17 billion in 2023, anticipated to increase to $53.78 billion. Large enterprises follow with $4.33 billion, which is projected to rise to $10.05 billion. The SMEs’ extensive adoption of Machine Learning for competitive advantage underpins their market dominance.
Machine Learning Market Trends and Future Forecast
Request a custom research report for industry.
Global Market Leaders and Top Companies in Machine Learning Industry
Google:
A pioneer in AI and Machine Learning technology, Google has integrated Machine Learning across its services, enhancing data processing capabilities and developing platforms like TensorFlow.IBM:
IBM is at the forefront of AI with IBM Watson, providing machine learning solutions tailored for various industries, enhancing decision-making and operational efficiencies.Microsoft:
Microsoft has invested heavily in AI technologies, incorporating machine learning into its Azure platform and services to help organizations harness data effectively.Amazon:
Amazon employs machine learning to optimize its e-commerce platform, enhancing customer experiences and operational efficiencies, while also offering ML services through AWS.NVIDIA:
NVIDIA specializes in GPU technology that accelerates machine learning processes. The company's hardware and software solutions are crucial for high-performance AI tasks.We're grateful to work with incredible clients.
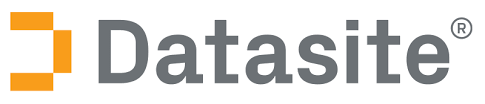
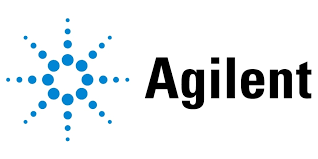

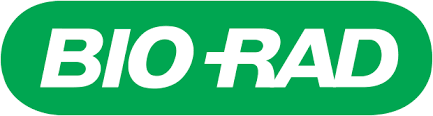
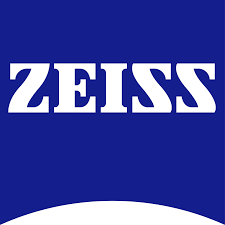
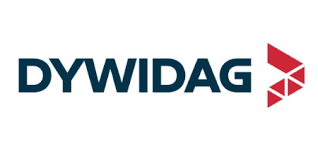
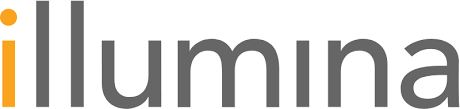
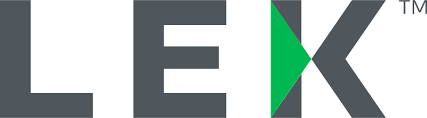
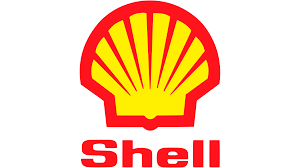
Related Industries
FAQs
What is the market size of machine learning?
The global machine learning market is valued at approximately $27.5 billion in 2023, projected to grow at a CAGR of 8.5%, reaching significant milestones by 2033.
What are the key market players or companies in the machine learning industry?
Key players in the machine learning market include tech giants such as Google, IBM, Microsoft, and Amazon, which dominate through their innovative ML services and strategies across various sectors.
What are the primary factors driving the growth in the machine learning industry?
The growth of the machine learning industry is primarily driven by increasing data volumes, advancements in AI technologies, demand for automation, and rising adoption across industries including healthcare and finance.
Which region is the fastest Growing in the machine learning market?
North America is currently the fastest-growing region in the machine learning market, with a projected market size of $24.50 billion by 2033, highlighting its strong tech infrastructure and innovation.
Does ConsaInsights provide customized market report data for the machine learning industry?
Yes, ConsaInsights offers customized market report data tailored to specific requirements within the machine learning industry, enabling clients to make informed decisions based on detailed analyses.
What deliverables can I expect from this machine learning market research project?
Clients can expect comprehensive deliverables including detailed market reports, trend analysis, competitor insights, and specific segment data to support strategic decision-making in the machine learning sector.
What are the market trends of machine learning?
Current market trends in machine learning include the rise of cloud-based deployment, increased investment in healthcare applications, and expanding use of supervised and unsupervised learning methodologies.