Predictive Maintenance In The Energy Market Size, Share, Industry Trends and Forecast to 2033
This report provides an in-depth analysis of the Predictive Maintenance market in the Energy sector, offering insights into current trends, market size, and forecasts extending to 2033, alongside strategic industry segmentation and regional performance evaluations.
Metric | Value |
---|---|
Study Period | 2023 - 2033 |
2023 Market Size | $15.60 Billion |
CAGR (2023-2033) | 7.2% |
2033 Market Size | $31.89 Billion |
Top Companies | GE Digital, Siemens AG, IBM, SAP SE |
Last Modified Date | 15 Nov 2024 |
Predictive Maintenance In The Energy Market Report (2023 - 2033)
Predictive Maintenance In The Energy Market Overview
What is the Market Size & CAGR of Predictive Maintenance In The Energy market in 2023?
Predictive Maintenance In The Energy Industry Analysis
Predictive Maintenance In The Energy Market Segmentation and Scope
Request a custom research report for industry.
Predictive Maintenance In The Energy Market Analysis Report by Region
Europe Predictive Maintenance In The Energy Market Report:
Europe's market is projected to grow from $4.17 billion in 2023 to $8.53 billion by 2033. The region's emphasis on digital transformation and sustainability initiatives, alongside legislative support for renewable energy, plays a crucial role in the expansion of predictive maintenance solutions.Asia Pacific Predictive Maintenance In The Energy Market Report:
In the Asia-Pacific region, the Predictive Maintenance market is anticipated to grow from $2.98 billion in 2023 to $6.10 billion in 2033. The rise of smart technologies and renewable energy investments in countries like China and India are critical growth drivers, encouraging a transition toward predictive maintenance systems.North America Predictive Maintenance In The Energy Market Report:
North America, leading the market, will see growth from $5.92 billion in 2023 to $12.11 billion in 2033, fueled by substantial investments in R&D and the integration of IoT and AI technologies in maintenance practices across various energy sectors.South America Predictive Maintenance In The Energy Market Report:
The South American market size is expected to increase from $1.35 billion in 2023 to $2.76 billion by 2033. This growth reflects the region's increasing focus on modernization within the energy sector, despite challenges in economic stability.Middle East & Africa Predictive Maintenance In The Energy Market Report:
In the Middle East and Africa, the market is expected to grow from $1.17 billion in 2023 to $2.39 billion by 2033. Increasing oil and gas output coupled with a shift towards more sustainable practices underscore the need for sophisticated predictive maintenance systems.Request a custom research report for industry.
Predictive Maintenance In The Energy Market Analysis Utilities
Global Predictive Maintenance in the Energy Market, By End-User Market Analysis (2023 - 2033)
Utilities represent the largest market segment, with a growth forecast from $6.30 billion in 2023 to $12.88 billion by 2033, maintaining a market share of 40.39%. Their adoption of predictive maintenance is driven by the need to optimize asset management and reduce operational costs.
Predictive Maintenance In The Energy Market Analysis Oil_and_gas
Global Predictive Maintenance in the Energy Market, By End-User Market Analysis (2023 - 2033)
The oil and gas sector is valued at $3.42 billion in 2023, expected to reach $6.99 billion by 2033, holding a consistent market share of 21.93%. Companies are leveraging predictive maintenance to enhance safety and uptime, a response to fluctuating international oil prices.
Predictive Maintenance In The Energy Market Analysis Renewable_energy
Global Predictive Maintenance in the Energy Market, By End-User Market Analysis (2023 - 2033)
The renewable energy segment is on a growth trajectory from $1.95 billion in 2023 to $3.98 billion by 2033, accounting for 12.49% market share. This growth is primarily driven by the increasing deployment of wind and solar energy systems globally.
Predictive Maintenance In The Energy Market Analysis Mining
Global Predictive Maintenance in the Energy Market, By End-User Market Analysis (2023 - 2033)
The mining sector, valued at $2.33 billion in 2023 and projected to reach $4.77 billion by 2033, holds a 14.96% market share. Predictive maintenance in this sector enhances equipment reliability and safety, addressing the increasing regulatory requirements for operational sustainability.
Predictive Maintenance In The Energy Market Analysis Manufacturing
Global Predictive Maintenance in the Energy Market, By End-User Market Analysis (2023 - 2033)
Manufacturing accounts for $1.60 billion in 2023 with a forecasted growth to $3.26 billion by 2033, representing a 10.23% share of the market. The utilization of predictive maintenance in manufacturing helps streamline operations and reduce production costs.
Predictive Maintenance In The Energy Market Analysis Hardware
Global Predictive Maintenance in the Energy Market, By Component Market Analysis (2023 - 2033)
Hardware segments dominate the market, with a size of $10.11 billion in 2023 expected to double to $20.67 billion by 2033, maintaining a share of 64.81%. The increasing integration of IoT sensors in energy systems is a primary factor driving hardware adoption.
Predictive Maintenance In The Energy Market Analysis Software
Global Predictive Maintenance in the Energy Market, By Component Market Analysis (2023 - 2033)
The software segment, valued at $3.56 billion in 2023, is projected to reach $7.28 billion by 2033, holding 22.82% of the market. Advanced analytics and AI developments are crucial in enhancing predictive maintenance software capabilities.
Predictive Maintenance In The Energy Market Analysis Services
Global Predictive Maintenance in the Energy Market, By Component Market Analysis (2023 - 2033)
Services are anticipated to grow from $1.93 billion in 2023 to $3.94 billion by 2033, yielding a market share of 12.37%. Consulting and maintenance services are becoming integral to the successful implementation of predictive maintenance systems.
Predictive Maintenance In The Energy Market Analysis Cloud_based_deployment
Global Predictive Maintenance in the Energy Market, By Deployment Type Market Analysis (2023 - 2033)
Cloud-based deployment is leading with a market size of $10.11 billion in 2023, expected to reach $20.67 billion by 2033, maintaining a dominant 64.81% share. The cost-effectiveness and scalability of cloud solutions are key to their widespread adoption.
Predictive Maintenance In The Energy Market Analysis On_premises_deployment
Global Predictive Maintenance in the Energy Market, By Deployment Type Market Analysis (2023 - 2033)
On-premises deployment is valued at $3.56 billion in 2023 and is projected to grow to $7.28 billion by 2033, representing 22.82% market share. Companies seeking greater control and security favor this deployment type.
Predictive Maintenance In The Energy Market Analysis Hybrid_deployment
Global Predictive Maintenance in the Energy Market, By Deployment Type Market Analysis (2023 - 2033)
Hybrid deployment, though smaller, is gaining traction from $1.93 billion in 2023 to $3.94 billion by 2033, with a share of 12.37%. This model appeals to industries requiring flexibility in their data management strategies.
Predictive Maintenance In The Energy Market Analysis Iot_solutions
Global Predictive Maintenance in the Energy Market, By Technology Market Analysis (2023 - 2033)
IoT solutions are crucial, with a market size of $10.11 billion in 2023 expected to double, reaching $20.67 billion by 2033 at a 64.81% share due to their ability to enhance data collection and analysis for maintenance predictions.
Predictive Maintenance In The Energy Market Analysis Data_analytics
Global Predictive Maintenance in the Energy Market, By Technology Market Analysis (2023 - 2033)
Data analytics is essential, growing from $3.56 billion in 2023 to $7.28 billion by 2033 and holding a 22.82% market share, largely driven by the increasing need for deeper insights and data-driven decision-making in maintenance.
Predictive Maintenance In The Energy Market Analysis Artificial_intelligence
Global Predictive Maintenance in the Energy Market, By Technology Market Analysis (2023 - 2033)
Artificial Intelligence is rapidly expanding, projected to rise from $1.93 billion in 2023 to $3.94 billion by 2033, capturing a 12.37% market share due to its transformative effects on predictive models and maintenance strategies.
Predictive Maintenance In The Energy Market Trends and Future Forecast
Request a custom research report for industry.
Global Market Leaders and Top Companies in Predictive Maintenance In The Energy Industry
GE Digital:
GE Digital offers comprehensive predictive maintenance solutions utilizing industrial IoT and big data analytics, leading the effort to enhance operational efficiencies in energy generation and distribution.Siemens AG:
Siemens AG provides sophisticated predictive maintenance software and services backed by analytics, enabling energy companies to optimize performance and reduce maintenance costs across various operations.IBM:
IBM's predictive maintenance solutions leverage its cloud and AI technologies to help energy providers minimize downtime and enhance service reliability through real-time data insights.SAP SE:
SAP SE delivers integrated predictive maintenance solutions tailored for the energy sector, utilizing machine learning and IoT technologies to transform maintenance operations.We're grateful to work with incredible clients.
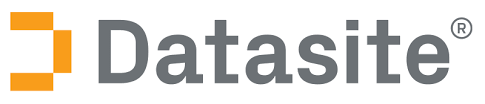
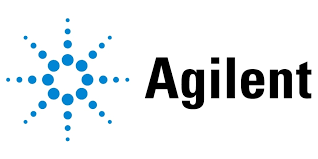

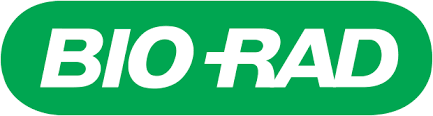
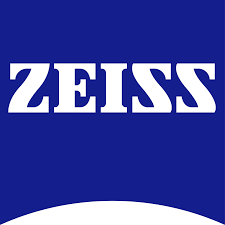
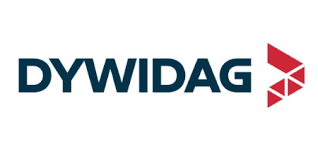
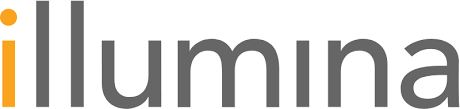
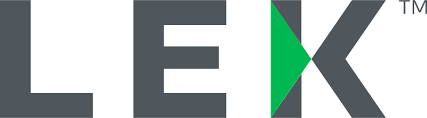
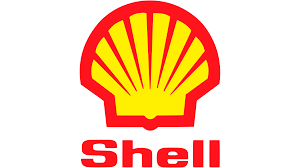