Recommendation Engine Market Size, Share, Industry Trends and Forecast to 2033
This report covers a comprehensive analysis of the Recommendation Engine market, highlighting key trends, insights, and data projections from 2023 to 2033, focusing on market size, growth rates, and competitive landscape.
Metric | Value |
---|---|
Study Period | 2023 - 2033 |
2023 Market Size | $8.00 Billion |
CAGR (2023-2033) | 15.0% |
2033 Market Size | $34.56 Billion |
Top Companies | Amazon Web Services, Microsoft, Google, IBM, Salesforce |
Last Modified Date | Invalid Date |
Recommendation Engine Market Report (2023 - 2033)
Recommendation Engine Market Overview
What is the Market Size & CAGR of Recommendation Engine market in 2023?
Recommendation Engine Industry Analysis
Recommendation Engine Market Segmentation and Scope
Request a custom research report for industry.
Recommendation Engine Market Analysis Report by Region
Europe Recommendation Engine Market Report:
The European market is projected to expand from $2.28 billion in 2023 to $9.84 billion by 2033. The region's focus on improving customer experience, coupled with stringent GDPR regulations necessitating better data management strategies, is boosting the adoption of recommendation engines across various sectors.Asia Pacific Recommendation Engine Market Report:
In the Asia Pacific region, the Recommendation Engine market is expected to grow from $1.52 billion in 2023 to $6.58 billion by 2033. The growth is driven by the rapid digital transformation across countries like China and India, where increasing internet penetration and mobile device adoption fuel e-commerce and online streaming services, demanding effective recommendation solutions.North America Recommendation Engine Market Report:
North America stands as a significant player with an expected market size growth from $2.78 billion in 2023 to $12.03 billion by 2033. The region, led by the U.S., showcases a robust IT infrastructure, high adoption of e-commerce platforms, and extensive use of data analytics, propelling the demand for advanced recommendation systems.South America Recommendation Engine Market Report:
The market in South America is anticipated to grow from $0.54 billion in 2023 to $2.35 billion by 2033. Despite being a smaller market, increasing adoption rates of technology and a growing interest in personalized customer engagement in industries such as retail and entertainment are key growth drivers.Middle East & Africa Recommendation Engine Market Report:
The Middle East and Africa market anticipates growth from $0.87 billion in 2023 to $3.76 billion by 2033. The growing digital economy and the push for enhanced customer analytics in the region's retail and hospitality sectors are key factors supporting this growth.Request a custom research report for industry.
Recommendation Engine Market Analysis By Product Type
Global Recommendation Engine Market, By Product Type Market Analysis (2024 - 2033)
The performance of different product types, such as Machine Learning, Deep Learning, and Collaborative Filtering, highlights the growing preference for AI-driven solutions. For instance, the Machine Learning segment is expected to grow from $4.93 billion in 2023 to $21.30 billion by 2033, maintaining the largest share in this domain.
Recommendation Engine Market Analysis By Deployment Mode
Global Recommendation Engine Market, By Deployment Mode Market Analysis (2024 - 2033)
Cloud-based solutions dominate the Recommendation Engine market, projected to grow from $7.11 billion in 2023 to $30.72 billion by 2033, reflecting the trend of scalable and cost-effective solutions adopted by businesses.
Recommendation Engine Market Analysis By Application
Global Recommendation Engine Market, By Application Market Analysis (2024 - 2033)
Applications in e-commerce stand out, expected to increase from $3.39 billion in 2023 to $14.64 billion by 2033, as companies look to enhance their online shopping experiences through personalized recommendations.
Recommendation Engine Market Analysis By User Type
Global Recommendation Engine Market, By User Type Market Analysis (2024 - 2033)
The businesses segment is forecasted to maintain a significant growth trajectory, expanding from $7.11 billion in 2023 to $30.72 billion by 2033. This growth indicates the demand for sophisticated recommendation systems across various industries to drive business outcomes.
Recommendation Engine Market Analysis By Technology
Global Recommendation Engine Market, By Technology Market Analysis (2024 - 2033)
Technologies like Machine Learning and Natural Language Processing are pivotal, with NLP set to increase from $0.97 billion in 2023 to $4.19 billion by 2033, showcasing the increasing need for context-aware recommendations.
Recommendation Engine Market Trends and Future Forecast
Request a custom research report for industry.
Global Market Leaders and Top Companies in Recommendation Engine Industry
Amazon Web Services:
AWS provides comprehensive machine learning services that enable businesses to integrate recommendation systems into their platforms, offering robust solutions with high scalability.Microsoft:
Microsoft's Azure platform offers advanced analytics and machine learning tools to build customizable recommendation engines, supporting diverse business applications.Google:
Google Cloud AI provides powerful recommendation systems leveraging extensive data analytics capabilities to improve user engagement and conversion rates.IBM:
IBM Watson offers personalized recommendation solutions, utilizing AI to enhance customer experiences across various industries, especially in retail and financial services.Salesforce:
Salesforce's AI-driven marketing tools integrate recommendation engines to help businesses personalize customer engagement, significantly improving marketing effectiveness.We're grateful to work with incredible clients.
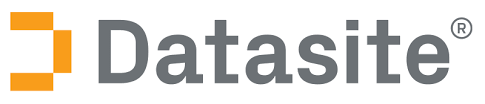
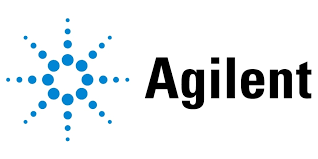

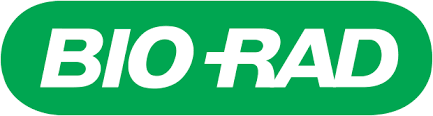
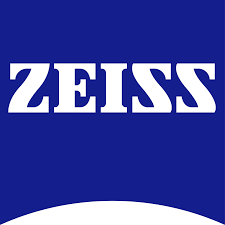
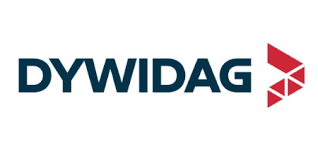
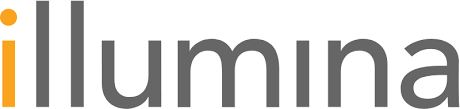
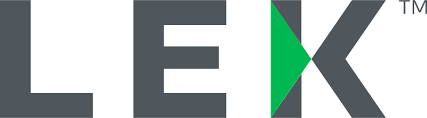
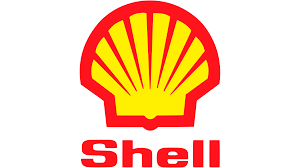
Related Industries
FAQs
What is the market size of recommendation Engine?
The recommendation engine market size is projected to reach $8 billion by 2033, with a robust CAGR of 15.0% from 2023. This growth is driven by increasing demand for personalized experiences in sectors such as e-commerce and media.
What are the key market players or companies in this recommendation Engine industry?
Key players in the recommendation engine market include leading tech companies and startups focusing on AI-driven solutions. These companies are innovating to improve algorithm efficiency and personalization capabilities, which is essential for maintaining a competitive edge.
What are the primary factors driving the growth in the recommendation engine industry?
The main drivers for growth include increasing data availability, advancements in machine learning, and the rising demand for personalized user experiences. E-commerce and media sectors are significant contributors to this trend, enhancing user engagement and sales.
Which region is the fastest Growing in the recommendation engine?
The fastest-growing region for the recommendation engine market is North America, projected to grow from $2.78 billion in 2023 to $12.03 billion by 2033. Asia Pacific also shows rapid growth, increasing from $1.52 billion to $6.58 billion in the same period.
Does ConsaInsights provide customized market report data for the recommendation engine industry?
Yes, ConsaInsights offers customized market report data tailored to specific needs within the recommendation engine industry. This allows clients to acquire in-depth insights and data relevant to their particular focus areas and business strategies.
What deliverables can I expect from this recommendation engine market research project?
Expected deliverables include comprehensive market reports, data analytics, segment analysis, regional insights, and strategic recommendations tailored to the recommendation engine market, equipping stakeholders with actionable insights for informed decision-making.
What are the market trends of recommendation engine?
Current trends in the recommendation engine market include a shift towards AI and machine learning technologies, increased focus on user privacy, and the integration of recommendation systems across various platforms, enhancing user interactions and personalization processes.